






Kickstart. Grow. Disrupt. Faster with AI-enabled team
Your journey to AI-powered engineering team starts here
Adopted by renowned, trusted, and leading enterprises





Effortless Engagement
AI-Powered Services
Your Ideas. Our Engineering. Limitless Possibilities.
Data Science
Unlock the power of your data with advanced analytics and machine learning to drive informed decisions.
Data Science
Unlock the power of your data with advanced analytics and machine learning to drive informed decisions.
Web Development
Establish a robust online presence with custom web applications tailored to your business needs.
Web Development
Establish a robust online presence with custom web applications tailored to your business needs.
UI/UX Design
Enhance user engagement through intuitive and visually appealing interface designs.
UI/UX Design
Enhance user engagement through intuitive and visually appealing interface designs.
Mobile Development
Reach your audience on the go with mobile apps optimized for performance and user experience.
Mobile Development
Reach your audience on the go with mobile apps optimized for performance and user experience.
DevOps / CloudOps
Streamline operations and ensure scalability with efficient DevOps and cloud solutions
DevOps / CloudOps
Streamline operations and ensure scalability with efficient DevOps and cloud solutions
Blockchain / Crypto
Explore innovative financial solutions with blockchain technology and cryptocurrency services.
Blockchain / Crypto
Explore innovative financial solutions with blockchain technology and cryptocurrency services.
Expert Collaboration
Seamless Team Integration
Scalable Engagement
Transparent Pricing, No Surprises
Flexible Plans for All
Experience a seamless journey from concept to launch, with fixed pricing and the flexibility to pause or cancel as needed.
Design
$1,499
/ 2 weeks
One request at a time. Pause or cancel anytime.
Unlimited requests
Senior design talent
24/7 access to Figma
Seamless project management
Continuous updates via your portal
Build, Test & Deploy
Popular
$7,999
/month
One request at a time. Pause or cancel anytime.
Everything in Design
Senior developer & devops talent
Full test coverage and code reviews
Testing & Quality Assurance
No outsourcing
Design
$1,499
/ 2 weeks
One request at a time. Pause or cancel anytime.
Unlimited requests
Senior design talent
24/7 access to Figma
Seamless project management
Continuous updates via your portal
Build, Test & Deploy
Popular
$7,999
/month
One request at a time. Pause or cancel anytime.
Everything in Design
Senior developer & devops talent
Full test coverage and code reviews
Testing & Quality Assurance
No outsourcing
We donate 2% of our profits to pediatric wellbeing

Trusted by Innovators Worldwide
What Our Partners Say
Hear from businesses who’ve transformed their products with our services
TXD created algorithmic trading platform and it was an absolute standout!
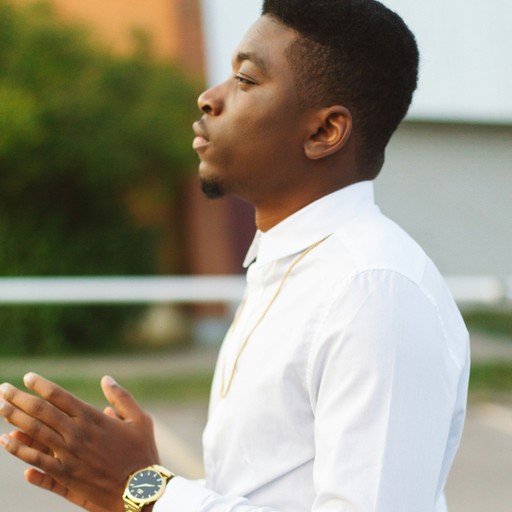
Alec Johnson
CSO of Capital Research Lab
TXD created algorithmic trading platform and it was an absolute standout!
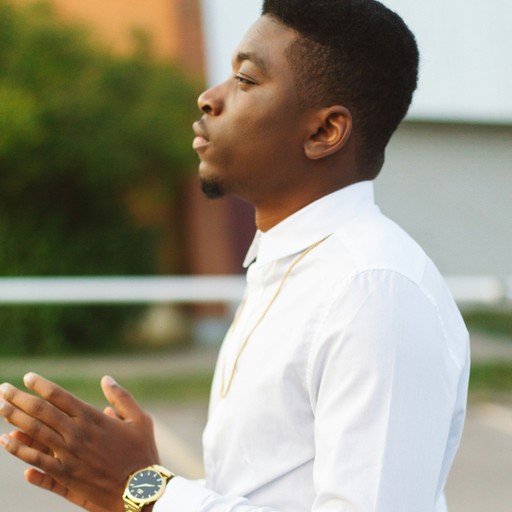
Alec Johnson
CSO of CRL
TXD created algorithmic trading platform and it was an absolute standout!
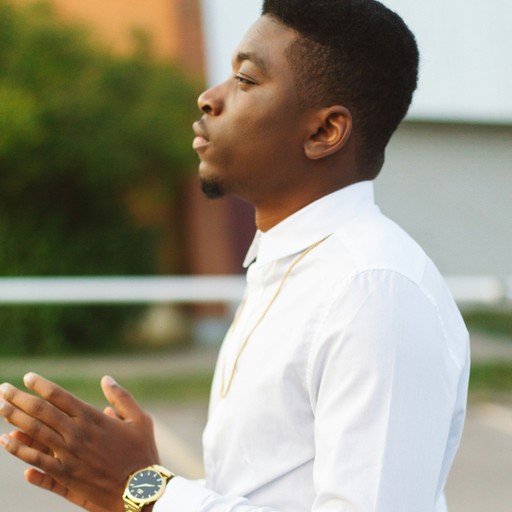
Alec Johnson
CSO of Capital Research Lab
A remarkable solution! It provides top-tier features, intuitive interfaces, and reliability
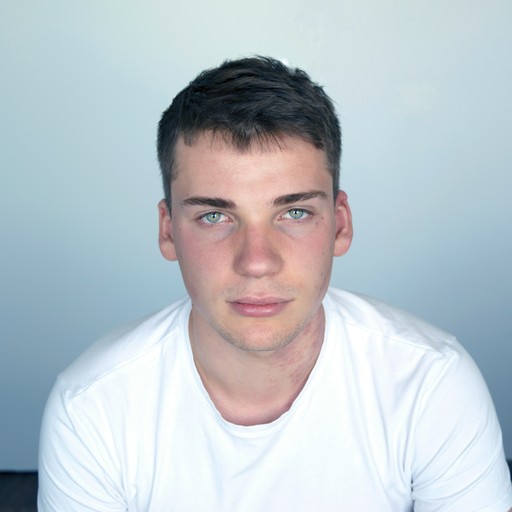
Joseph Clough
Hypnosis
A remarkable solution! It provides top-tier features, intuitive interfaces, and reliability
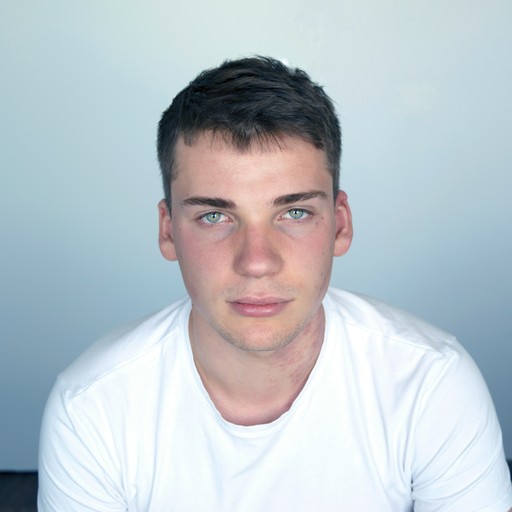
Joseph Clough
Hypnosis
Recognises the professionalism and problem solving skills that the TXD team has.
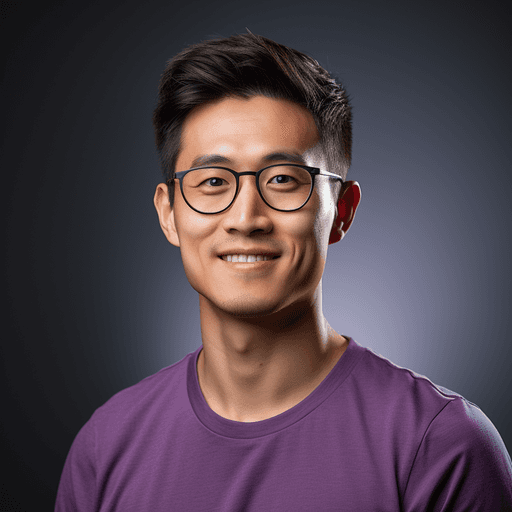
Tim Aballo
Arbitrage Ops
Recognises the professionalism and problem solving skills that the TXD team has.
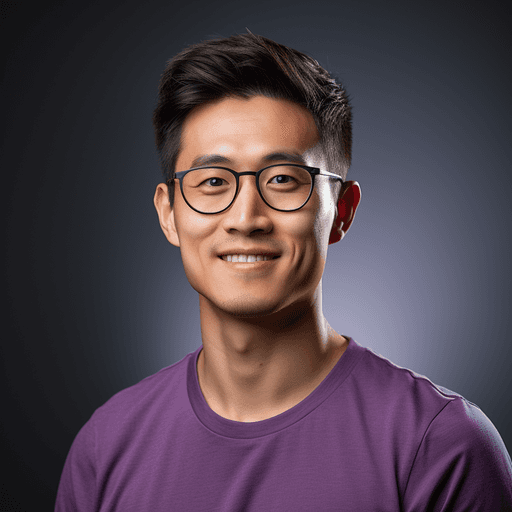
Tim Aballo
Arbitrage Ops
We found Txend to be always one step ahead in terms of identifying and solving problems.
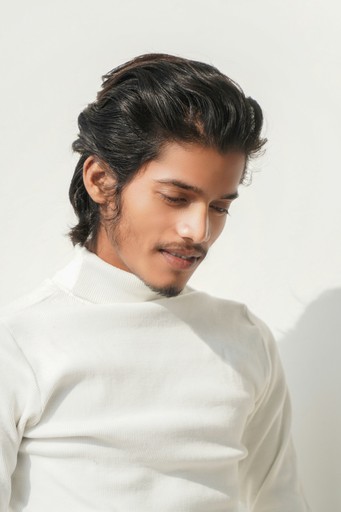
Abid Faiz
Levis Straus
We found Txend to be always one step ahead in terms of identifying and solving problems.
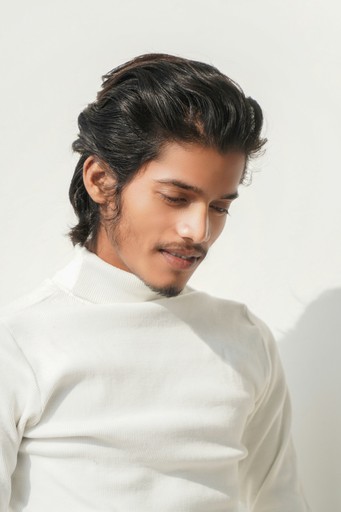
Abid Faiz
Levis Straus
The teams are talented and regularly make that extra effort to achieve results on time
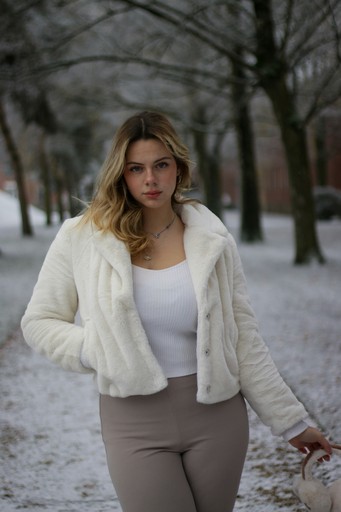
Mike Marinos
Point Duty
The teams are talented and regularly make that extra effort to achieve results on time
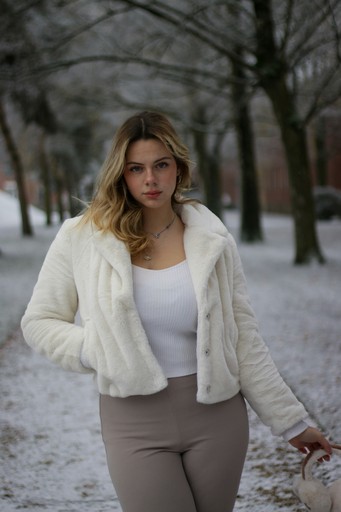
Mike Marinos
Point Duty
I was very impressed by how they managed the development and the difficulties that arose.
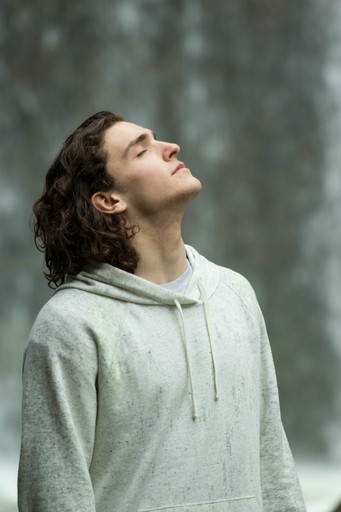
Zachary Gressmann
Founder, Avant Garde Tech
I was very impressed by how they managed the development and the difficulties that arose.
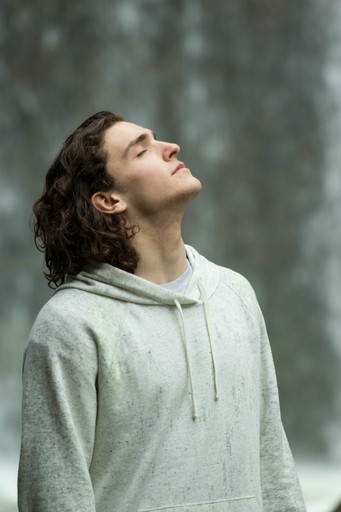
Zachary Gressmann
Founder, Avant Garde Tech
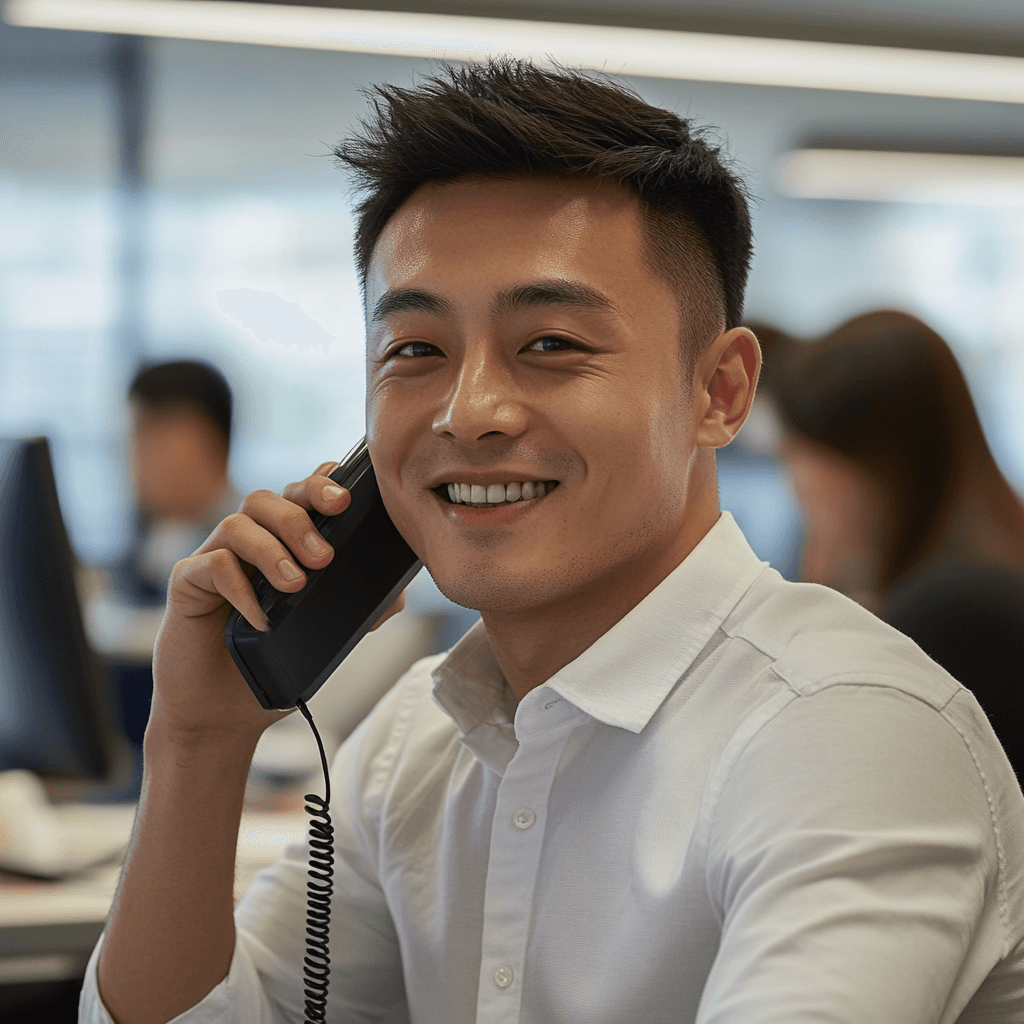

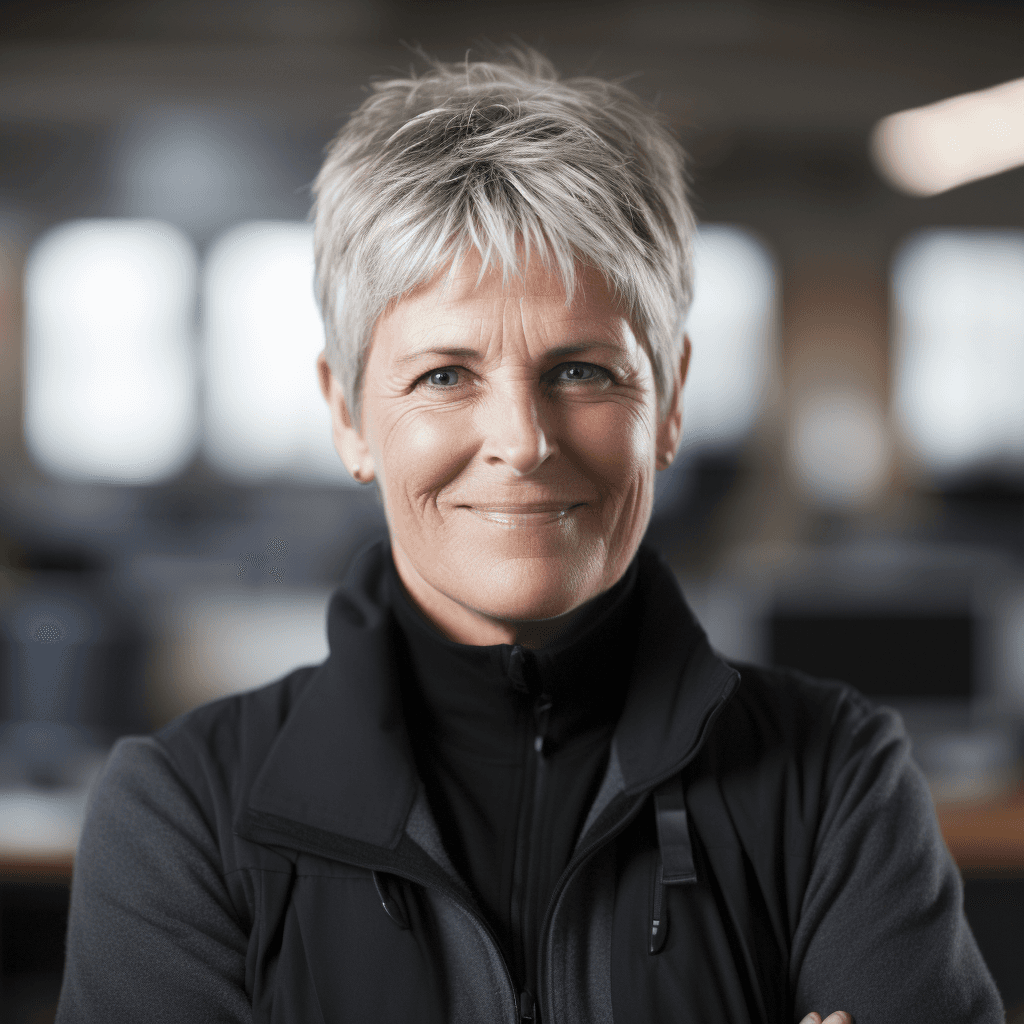
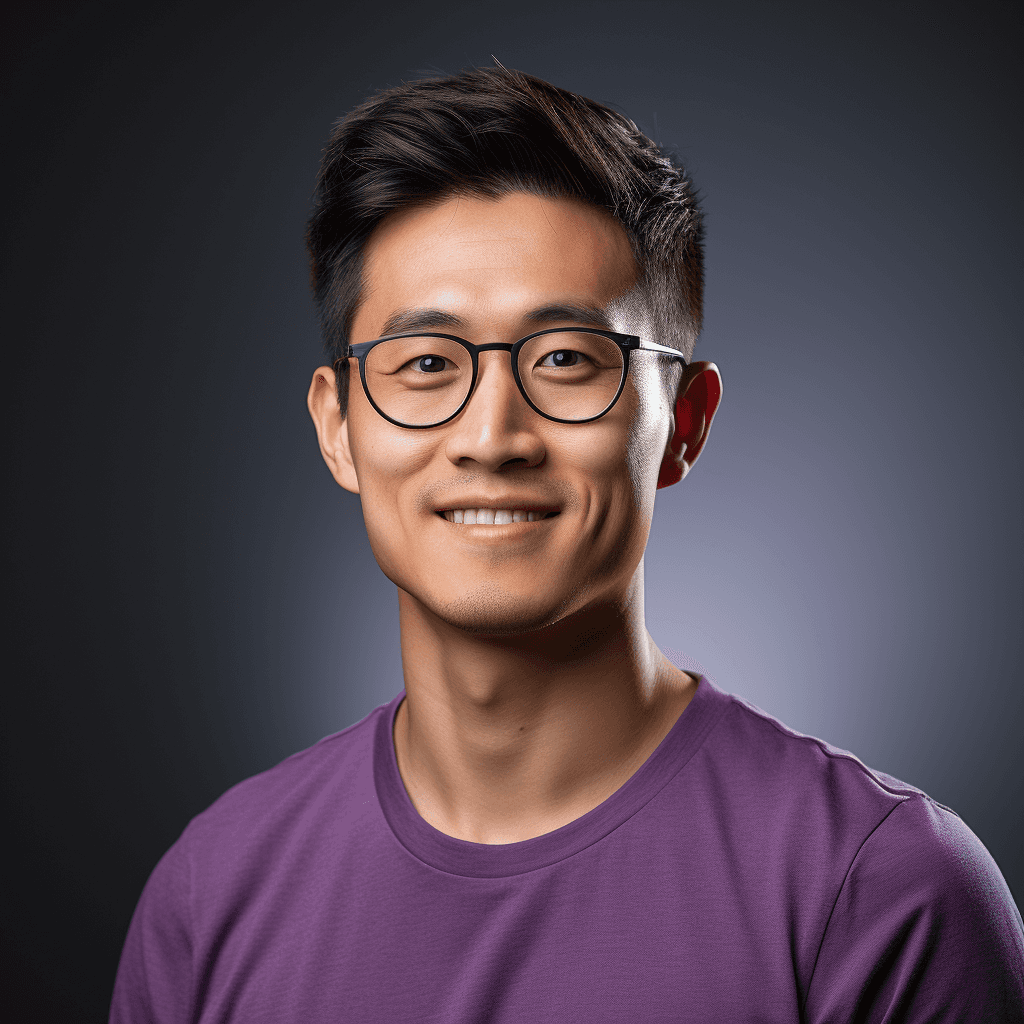
Trusted by
+
innovators worldwide

Your Queries, Simplified
Questions? Answers!
Find quick answers to the most common questions about our platform
What is Txend ?
Txend Inc is an elite full-cycle AI-enabled software development company with a world-class team of talented data scientists, developers, designers, mathematicians, engineers, and creative artists.
How fast can Txend deliver a digital product?
Delivery time depends on your project's complexity and needs. With our agile team, we usually go from idea to market-ready product in just a few weeks.
Which package best fits my project?
The right package depends on your project’s scope and business needs. The Design package suits Innovators looking to refresh their app’s look or launch something new, while the Build and Test package is ideal for ongoing projects needing continuous development, testing, and design.
Can I cancel or pause my subscription anytime?
Yes, our flexible subscription lets you pause or cancel anytime, giving you full control over your project and budget.
Are there any extra costs beyond the subscription fee?
All potential costs are discussed upfront during the discovery phase. Our subscription typically covers all agreed services with no hidden fees. However, you're responsible for any external costs like third-party tools, hosting, or special licenses needed for your project.
How often will I receive updates about my project?
You'll get regular updates through your client portal. We can schedule weekly or bi-weekly meetings, or provide asynchronous updates, based on your preference.
What technologies does Txend specialize in?
For design projects, we typically use Figma and Adobe Suite, tailored to your needs. Our skilled team works with a variety of modern technologies. For most projects, we recommend React (or React Native), Node.js, TypeScript, Mongo, PostgreSQL, Redis, and cloud platforms like AWS to build scalable, high-performance applications.
How does Txend protect the security of my project?
As an ISO 27001 compliant company, we take the security of your project seriously by employing a comprehensive approach. This includes conducting regular security audits to identify potential vulnerabilities, implementing data encryption to protect sensitive information, and following secure coding practices to minimize risks. Our team also adheres to strict confidentiality agreements and operates under a clear commitment to safeguarding your data. Furthermore, we use advanced security tools and maintain continuous monitoring to ensure that your project remains protected at every stage of development.
Feel free to mail us for any enquiries : discover@txend.com
Your Queries, Simplified
Questions? Answers!
Find quick answers to the most common questions about our platform
What is Txend ?
How fast can Txend deliver a digital product?
Which package best fits my project?
Can I cancel or pause my subscription anytime?
Are there any extra costs beyond the subscription fee?
How often will I receive updates about my project?
What technologies does Txend specialize in?
How does Txend protect the security of my project?
Feel free to mail us for any enquiries :
discover@txend.com
Trusted by 10,000+ businesses
Build AI-Enabled Engineering Now!!
Scale Smarter: Hire Teams that Build with AI. From Idea to Impact—Faster with AI.